Rome – October 7, 2024
As the reach of AI expands, experts are focusing on the essential elements that ensure the success of AI projects. Experts agree that data quality is the fundamental factor that affects the performance of AI systems. The development and refinement of AI applications depend on high-quality data, which affects the accuracy, reliability, and fairness of AI model outputs.
The "learning" that occurs in deep learning AI systems is directly related to the data used to "train" and "teach" these AI systems. High-quality data is essential to the development and refinement of AI applications because it directly affects the accuracy, reliability, and fairness of the outputs from AI models. A review of best practices shows that AI models with intentionally proactive, high-context data management strategies (such as those executed within TranslationOS) tend to outperform AI models with relatively limited, static data management practices typical of traditional TMS systems.
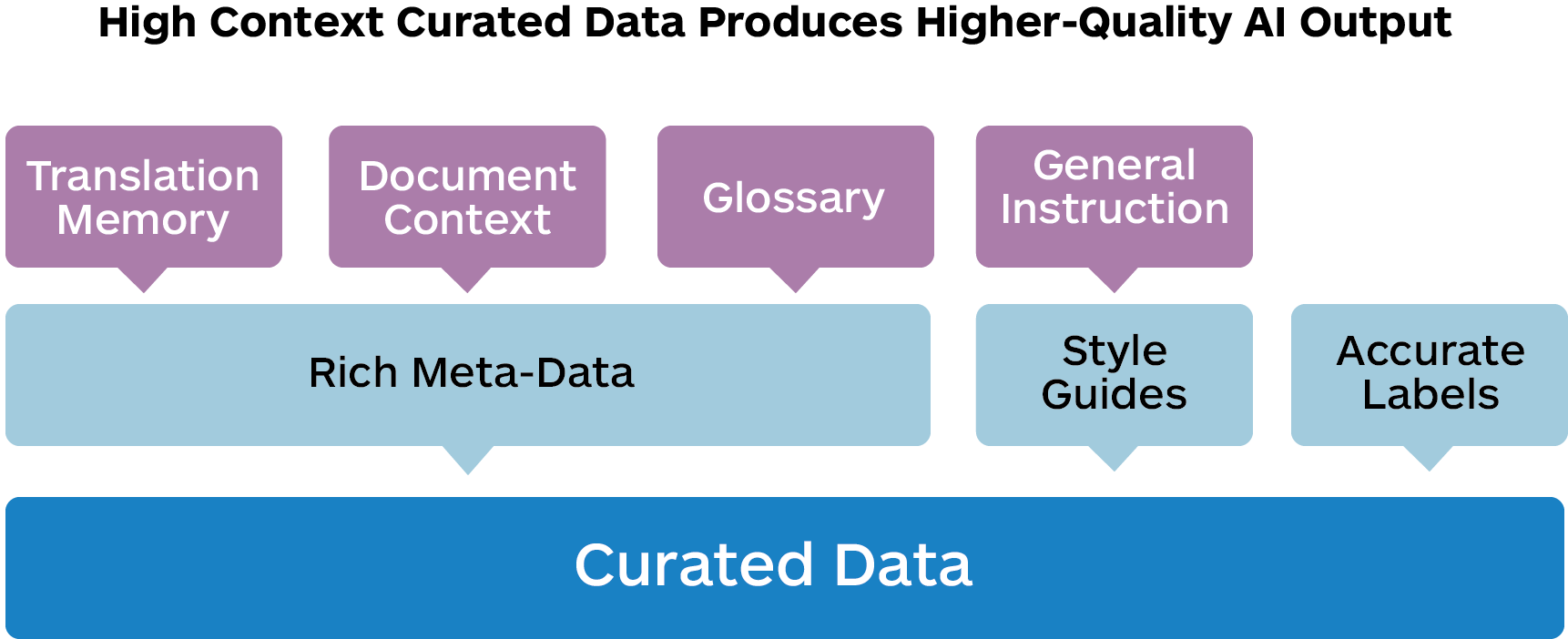
The benefits of an active data quality orientation can be summarized in its likely impact and some best practices below.
The Impact of High-Quality Data in AI
- Improved Accuracy and Efficiency: High-quality data enhances the accuracy of AI predictions and decisions. AI models can interpret and analyze new information more accurately and produce better output.
- Reliability and Stability: High-quality data ensures that AI systems are stable and perform consistently.
- Bias Reduction: Ensuring AI systems are trained on diverse and representative data helps mitigate biases in AI outputs and produces useful outcomes in production settings.
Best Practices for Ensuring Data Quality
- Data Governance: Implementing robust frameworks is essential to maintain data quality. This includes data verification, cleansing, and regular audits to identify and address quality issues.
- Continuous Improvement: AI models should be updated with new and accurate data to reflect real-world scenarios accurately. This involves continuous learning and regular retraining to ensure models remain robust over time.
- Ongoing Data Curation: The ability to identify and capture the most relevant and useful new data once an AI model is put into production is perhaps the most important driver of superior model performance. This increasingly means providing more contextual and stylistic information to the AI, and richer metadata in addition to basics like translation memory. The careful collection and redeployment of real-time corrective feedback in addition to the maintenance of existing data assets is a critical data management task necessary to enable and drive continuing model evolution.
When we consider Language AI applications focused on optimizing the language translation task, we find that three types of data are important:
- The training data that is used to build the foundational baseline model. Historically this has focused exclusively on translation memory assets.
- The incremental use-case or client-specific data that is used to fine-tune and adapt the baseline model to perform optimally for different use cases. Again, historically this has had a primary focus on enterprise translation memory. With changes in the AI technology capabilities new data is needed to provide accurate contextual information to the AI, and active experimentation shows that providing rich contextual information produces more precise and nuanced outputs that are much closer to desired results.
- Ongoing corrective feedback and selective strategic data creation that further refine and improve AI model performance in production scenarios. This fresh data is valuable in aligning and fine-tuning the AI system to produce better outputs for the most recent and current projects.
These three types of data have the potential to enhance and improve language translation AI. The most successful practitioners learn to collect, clean, maintain, and use all three types of data to address real-world business use cases. Neural MT experts have been working with all three types of data for many years now. However, in the localization world, most of the focus has been on the second type (customer translation memory), which is used to customize or adapt large generic (static) models. Most often unsuccessfully.
It is also evident that expert human translators will frequently utilize supplementary contextual information, such as style guides, glossaries, corporate tone guidelines, and general instructions regarding the intended use of the content. This information, when properly curated, can also be supplied to AI to enhance the quality of the results.
As we head more deeply into the world of LLM translation models the focus shifts much more to the data that is used to adapt and tune foundation models either through simple prompt-based translation requests or more sophisticated RAG (Retrieval-Augmented Generation) and other advanced techniques. RAG is the process of optimizing the output of an LLM model so that it references an authoritative knowledge base (typically, contextual translation memory in this case) outside of its training data sources before generating a response. It is possible to provide an LLM a richer data set to ensure that outputs are well aligned with specific and nuanced requirements.
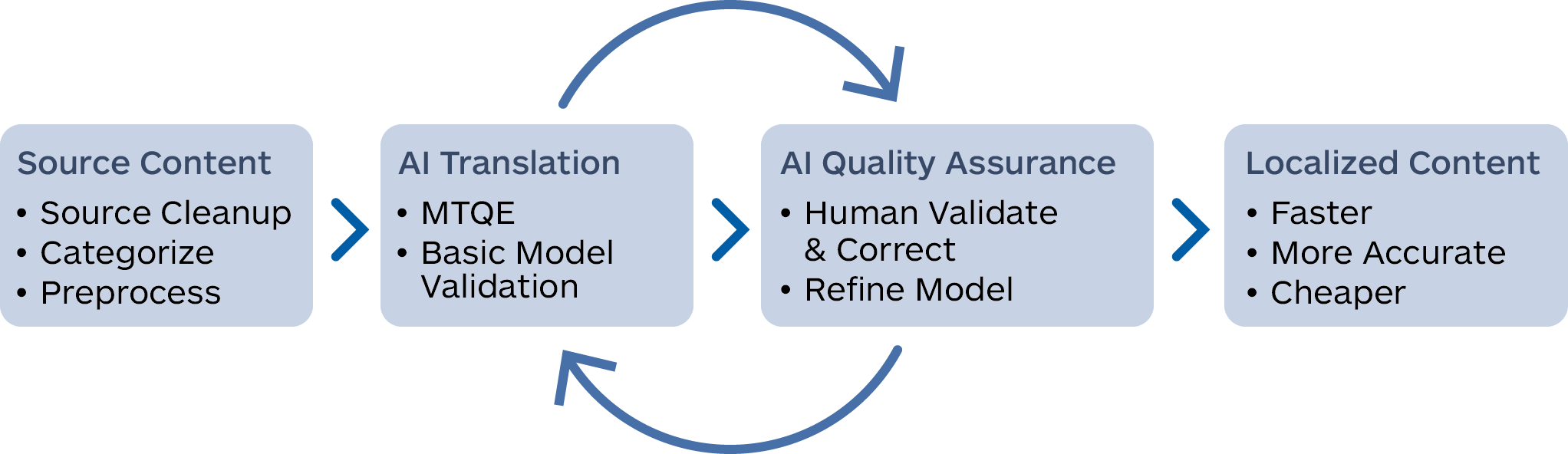
While simple TM based strategies can often provide useful results, a different mindset and approach are needed to achieve production efficiency and deployment at an enterprise scale. Automation, throughput, and efficiency are requirements for any dynamic enterprise setting and simplistic and naive prompt-based approaches are not likely to scale effectively. These simplistic approaches are also unlikely to be viable in a production setting where automation is a key requirement.
Legacy Translation Production Models are Not Designed to be AI-First
Existing translation production models used in localization often result in sub-optimal outcomes in the emerging AI-first world as these legacy production models have historically evolved from a heavy focus on project management and inter-agency coordination needs that are required in scenarios where multiple LSPs are working independently.
Thus, they are optimized for project management and coordination between multiple agencies that may have unique and different production processes that are not easily aligned. In many cases, the data assets of each of these agencies are likely to be incompatible or difficult to consolidate at both a technical and logical level because of the different tools and production processes that are being employed. This results in ongoing inefficiencies, persistent errors, and inconsistencies that require constant oversight and cleanup.
The data sharing between these independent agencies is limited and thus robust, overall enterprise-level data integrity is difficult to accomplish because of tool and process incompatibilities. This legacy approach does not align well with the AI-first mindset which by definition is very data-centric and requires that data assets have an overall consistency, be open, be easily leveraged across different processes, and easily interact with new AI-powered tools.
Many legacy TMS systems constrict and confine access to potentially valuable translation memory and other linguistic assets that could be useful to the new Linguistic AI-powered tools and processes. Data sharing across TMS and current adaptive MT platforms is primitive and most of these systems are not flexible or open enough to make the transition to an AI-first platform.
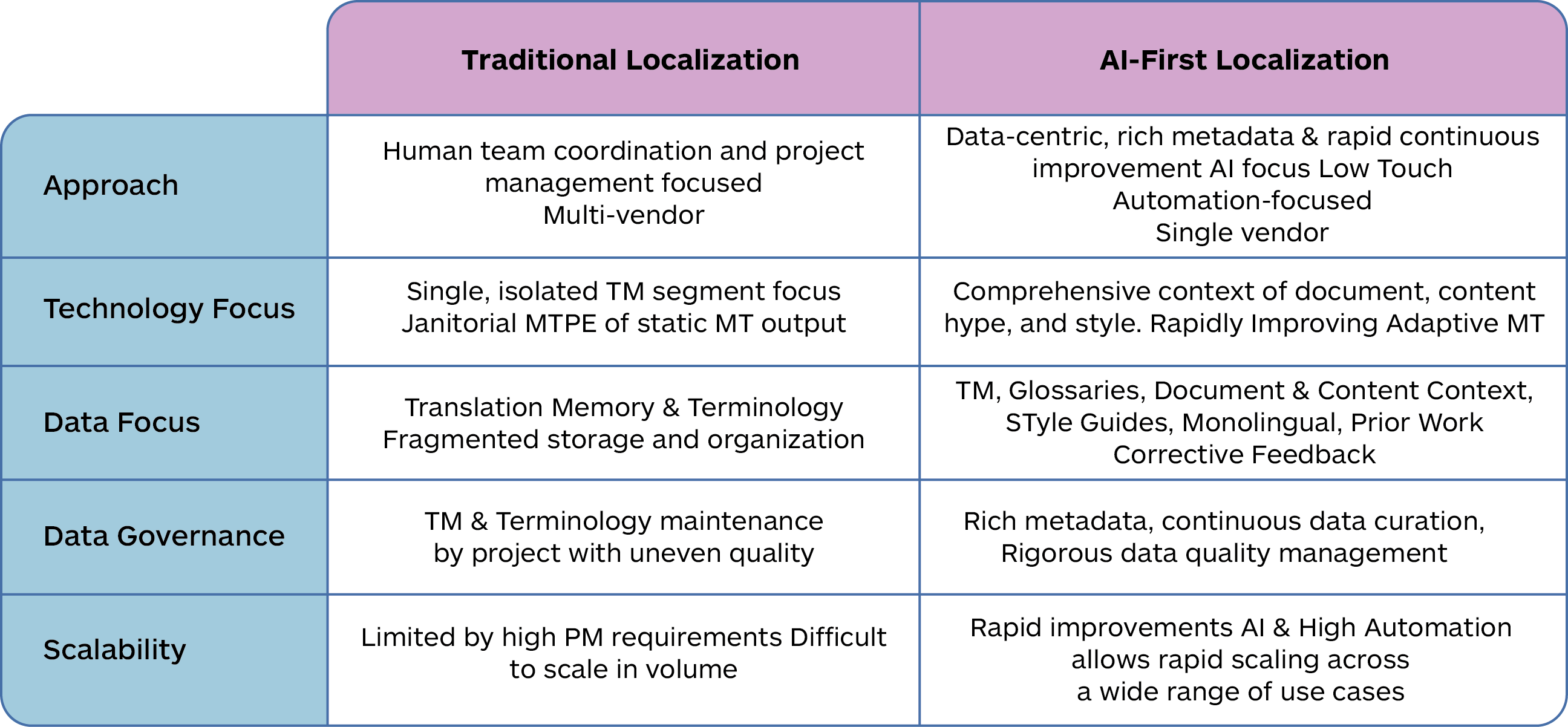
Another major benefit of AI-first approaches is that they allow much deeper personalization of an enterprise's unique needs. This allows use-case-specific adaptation to handle both structured enterprise content and unstructured user-generated content equally well. Traditional localization by definition is limited to what generic approaches will allow.
The single-segment TM-centric approach represents a significant challenge for legacy tools in effectively embracing AI technologies. Translation memories store previous translations and are retrieved for similar segments in new projects. However, they lack metadata and do not adapt to new contexts or learn from feedback unless manually updated. The majority of legacy tools focus on a single TM segment in isolation. In the typical translation production process, translators only see unmatched segments that need to be translated and have limited awareness of the larger document context. This fragmented view of the overall context is the cause of many inconsistent and erroneous translations. This is further compounded in multi-vendor scenarios where translators may adhere to completely different practices and have limited inter-agency communication.
Note how a sentence in isolation, without context, could make it challenging to understand the context and proper treatment of the word “pitch” in the following examples.
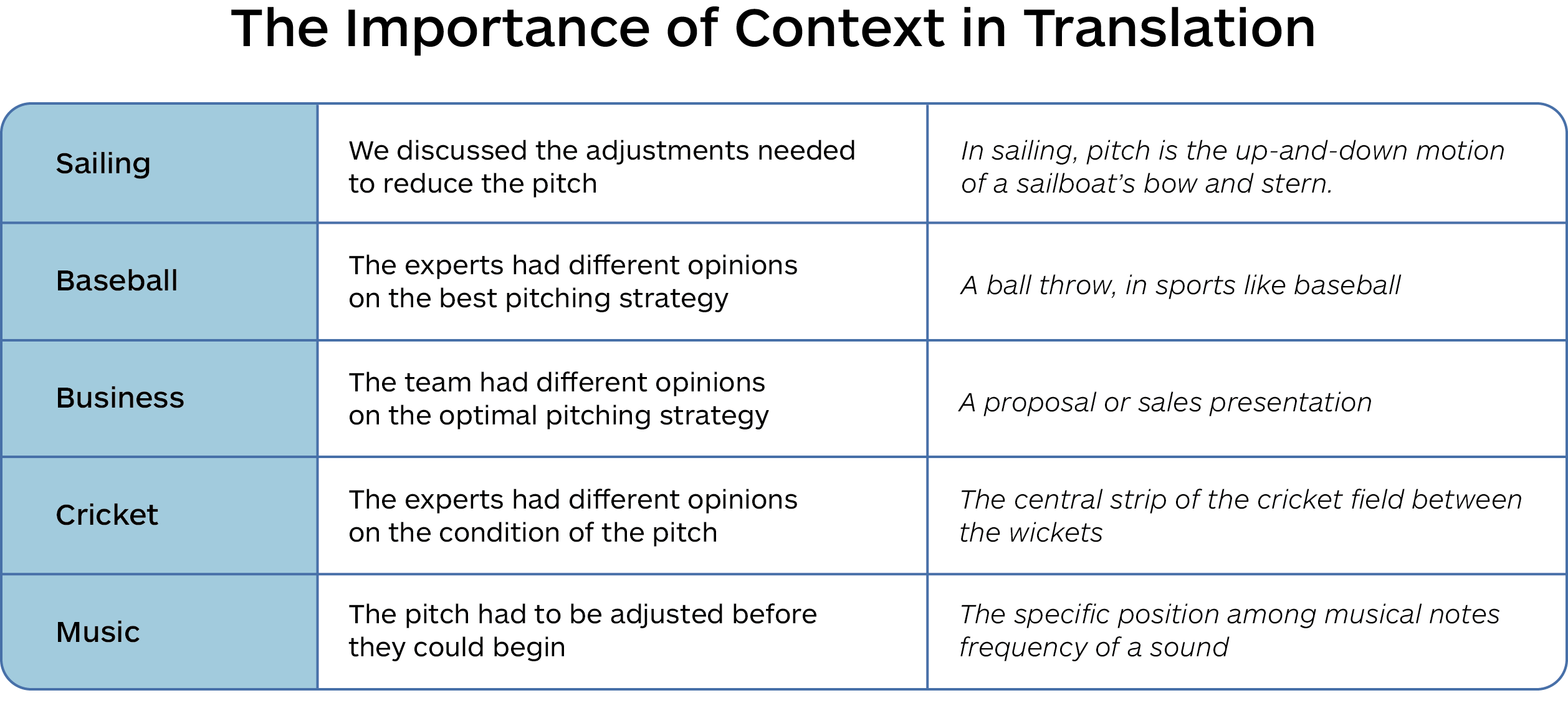
Another way to look at this is that traditional localization approaches focus only on Lexical Search which provides structure and precision by delivering exact keyword matches. An AI-first approach uses both Lexical and Semantic Search and thus is more likely to understand synonyms and the deeper context of queries or individual content strings, therefore more capable of uncovering the user’s true intent. In fact, it is even possible to provide additional context that captures style, tone and updated communication preferences depending on the available metadata.
Legacy systems overly concentrate on isolated translation segments, neglecting the wider document context and stylistic subtleties. This results in inefficiencies, inconsistencies, and avoidable errors, particularly in multi-vendor environments, necessitating substantial corrective and supervisory actions. Accessing and sharing TM data with other AI tools is also difficult due to designs optimized for segment matching within a single proprietary system.
In contrast, an AI-first approach within TranslationOS is designed to continuously learn and adapt, comprehending the specific context and tone of documents, and incorporating previous corrections. The dynamic and flexible curated data infrastructure of TranslationOS can be repurposed with new Language AI technology, swiftly delivering quality improvements and cost reductions to emerging technologies. Thus, innovations like Trust Attention derived from carefully curated data carry their benefits forward as new AI technology is incorporated. The latest AI outputs produced with the latest AI technology can improve daily with minimal management, and the more they are used, the faster the quality and efficiency are enhanced.
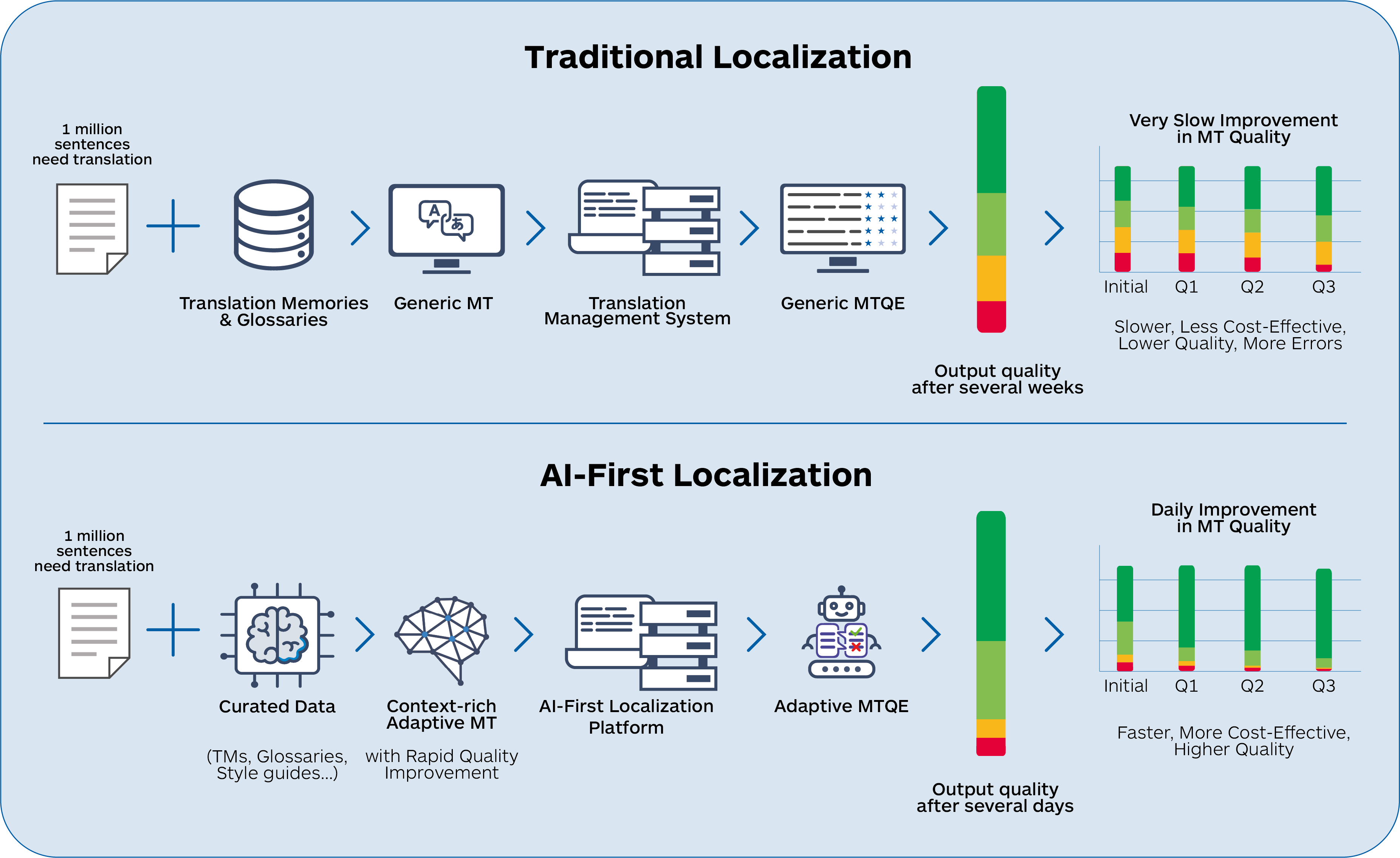
More importantly, new AI approaches that involve fine-tuning can utilize a richer data set to produce better AI output. However, this is only possible if the translation memory and other linguistic data used have rich metadata to easily bring the “right” relevant data to the AI and critical data is not locked in a proprietary TMS bin.
Data centricity requires a common and consistent data architecture and rich metadata that allows the data to be optimally used across all of the following processes in an integrated way:
- Source data cleanup and pre-translation preparation
- Management, maintenance, improvement, and ongoing cleaning of TM assets
- Automated translation using the most relevant existing TM and terminology
- Efficient monitoring and collection of data related to the refinement of machine output to immediately improve the workflow by making relevant and needed modifications in upstream processes and continually improve overall production efficiency and quality outcomes
- Rating and categorization of information of human translator resources to enable optimal selection by competence, specialization, productivity, and prior performance
A data-centric culture would facilitate ongoing improvements in the quality and quantity of highly relevant, validated data, which can be used to enhance AI models. It would also support an increasing range of addressable use cases and encourage multilingual content sharing on a larger scale.
The following example shows how even a single sentence of contextual information can inform and improve an automated translation (by upcoming LLM-based MT Lara ) immediately. In enterprise settings there would be multiple kinds of context that could be given to the system to allow greater precision.
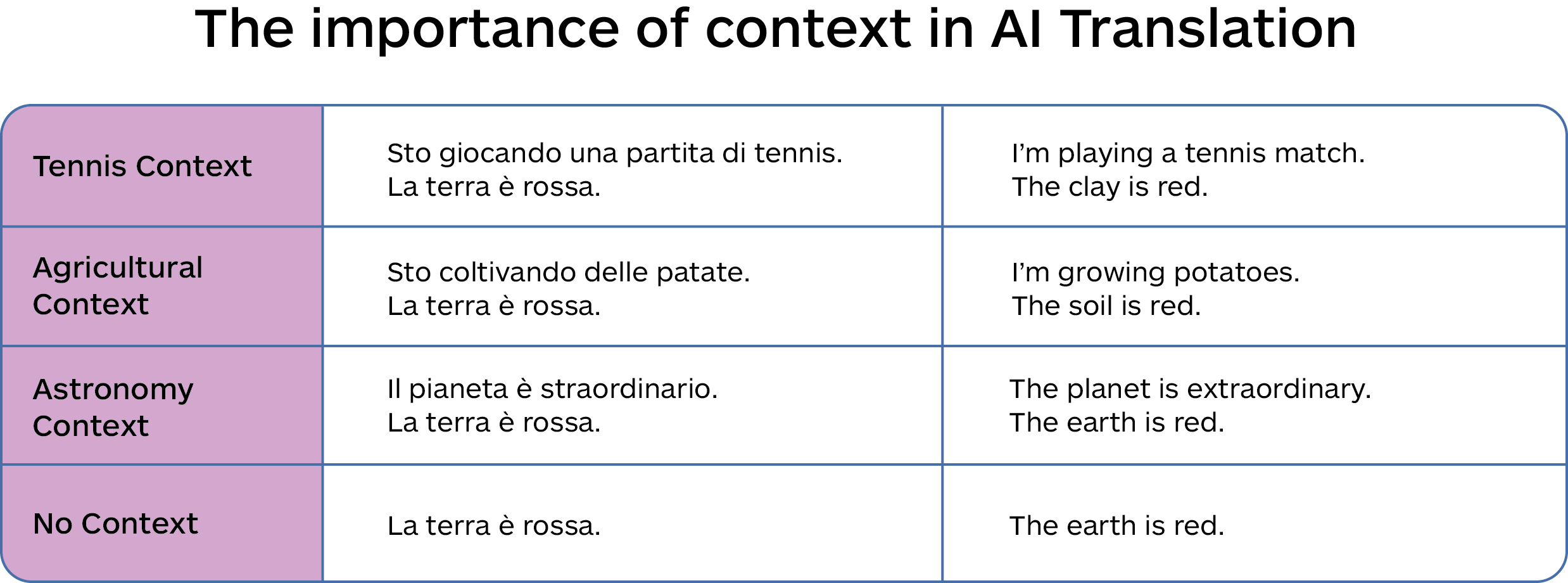
What do we mean by AI-First Localization?
AI-first localization technology refers to the integration of artificial intelligence into the localization process to enhance translation and cultural adaptation of content such as product information documents, apps, software, websites, and more.
An AI-first workflow guarantees that the AI is receiving all relevant information needed to produce optimal output and includes most if not all the curated data described previously. This can include style guides, general instructions, previous corrective feedback on similar projects, labels, and even general instructions in a machine-digestible format.
To build a strong data curation infrastructure, it's crucial to know what the "right data" is for improving AI outcomes. Interestingly, the same contextual and background situational data that expert translators find useful is also valuable for AI systems. Simply having access to a translation memory is not sufficient; access to other contextual data is necessary.
Gathering this comprehensive contextual data set and presenting it to the AI in an appropriate AI-ready format is at the core of data curation work. The more detailed and relevant the contextual information provided to AI, the better the AI output. However, this needs to be done efficiently to avoid overly large inputs, especially in high-volume use-case environments.
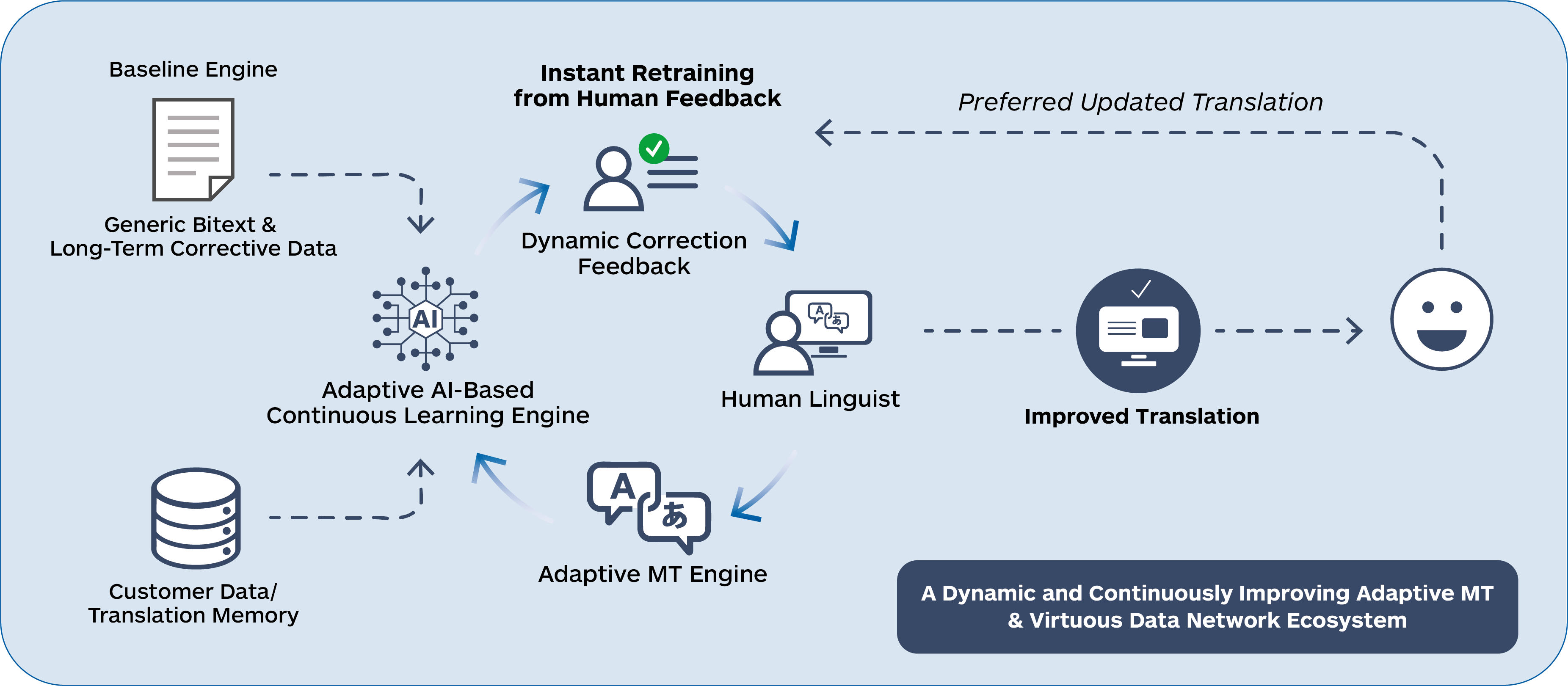
In typical legacy localization scenarios, data tends to get stale, and inconsistent, and becomes a cause for inefficiencies and sub-optimal results. The data used to steer AI needs regular maintenance to ensure ongoing production efficiencies.
An AI-first approach is designed to evolve and learn from both ongoing corrective feedback, and process adjustments that are made during regular production work. More data is good, but more high-quality data is better.
Key characteristics of the AI-first approach include:
- Rich metadata for all linguistic assets so the right data can easily be redeployed where needed
- Data-driven approach leveraging large high-quality datasets and continuously improving machine learning
- Context and instruction-rich data inputs to ensure optimal AI system outputs
- Production work that feeds into an expanding store of curated data. Implementing rapid error detection and correction processes within workflows produces a consistent flow of fresh, high-quality, verified data for continuous model improvements
- Automation of repetitive tasks and workflows that focus on managing by exception when the system is slightly out of alignment rather than requiring oversight of all machine output
- Ability to configure the human-to-machine interface differently for different tasks to ensure efficiency. This can range from pre-analysis of source data, real-time collaborative data exchange, adaptive MT model steering, automated quality estimation, and automated post-editing
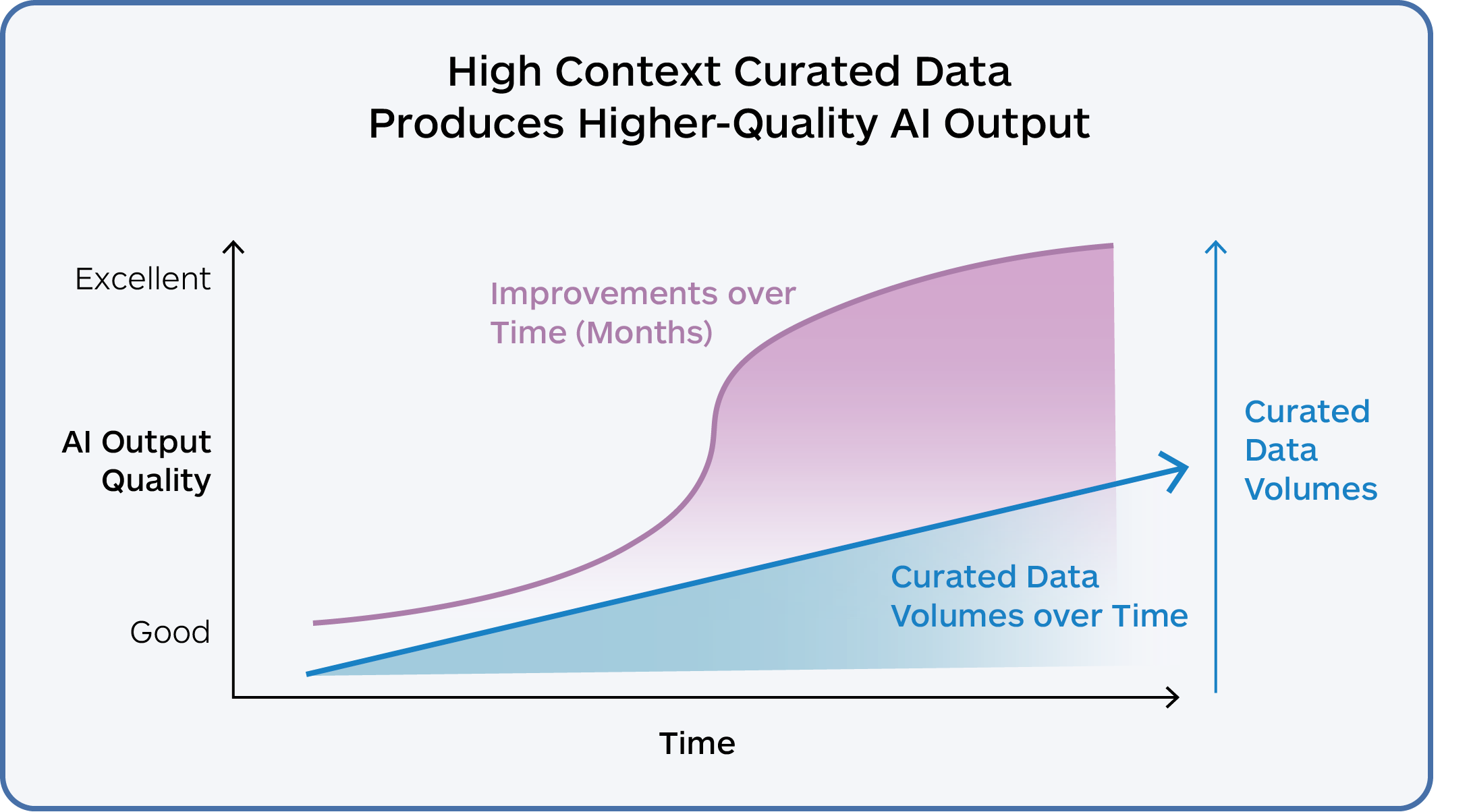
- Balance automation with continuing expert human feedback and adjustments
- Continuously monitor, refine and improve AI models and processes
- Implement robust data management, maintenance and new high-quality data acquisition strategies
- Foster collaboration between AI systems and human linguists by providing meaningful assistive feedback in production
Other components of the AI-first technology stack include:
- Adaptive, rapidly improving machine translation (NMT or LLM MT) systems
- Natural language processing (NLP) capabilities for other linguistic tasks
- Machine learning algorithms that can be applied to different types of production data for continuous improvement
- AI-powered quality assurance and consistency checks that can be improved and upgraded
- Translation memory and terminology management systems
- Workflow automation and project management tools
- Data analytics and predictive modeling capabilities
- APIs for seamless integration with other systems
- Generative AI for Multilingual Content Creation
- Cloud-based platforms for scalability and accessibility
Some of the key elements needed are described below in more detail:
Data-Centric Culture: This focus extends beyond the linguistic asset data like TM, terminology, and glossaries. As we move further into AI-powered production the data will also include more document and content-relevant contextual data, accurate labeling, relevant monolingual data that can improve fluency and stylistic adaptation, style guides and enterprise tone-related data. And of course, rich metadata so that the right data can be assembled and input when needed.
All this data is not static and is continuously being improved and cleaned as ongoing production use reveals flaws and problems with legacy data stores. There is a constant quality curation going on with the data which results in constantly improving output.
AI-first also requires that workflow systems acquire and collect new, fresh data continuously and add to the legacy stores as ongoing corrective feedback is provided in production. AI-first scenarios demand a data-focused process that continues to refine and improve the core data assets that drive current and future AI processes.
In addition to these evolving linguistic assets, there is also a need to build improving data descriptions on the human resources that are interacting with the linguistic data. Thus, having detailed profiles on the quality, capabilities, specialization, and competence of human translator resources (e.g. T-Rank™ ) is also critical to designing optimal workflows that are deeply aligned with different client requirements. It is increasingly understood that a close and dynamic relationship with expert human oversight is possibly the single most powerful contribution to continually better system performance.
Open access to Data Assets with new Language AI tools & LLMs: The linguistic assets in particular need to be easily redeployed and connected to new Linguistic AI based tools as these improvements emerge and transform the production efficiency of legacy workflows. The lack of openness and straightforward access to legacy linguistic data assets create a situation characterized as “tech-debt”. Legacy TMS systems are optimized for workflows that emphasize project management and project coordination but have little to no understanding or ability to support new AI processes that need access to specific subsets of linguistic data to perform optimally. Unfortunately, many legacy TMS systems used to manage linguistic assets create many technical obstacles with straightforward data sharing and exchange resulting in expensive technical debt-laden complexity that undermines production efficiency. The problems created by tech debt include many of the following:
- Systemic instability and failures
- Frequent outages and downtime
- Security vulnerabilities
- Reduced developmental agility and velocity
- Significantly higher maintenance costs and complexity
- Lower data asset quality that is unable to evolve and improve
- Loss of competitive advantage
- Reduced innovation and limited ability to introduce new innovative features
- Poor User Experience due to slow or error-prone functionality
The AI-first world demands open and easy access to legacy data that can provide useful input to new Linguistic AI processes and needs a robust foundational organization so that this can be done efficiently with minimal friction. This is also why it often makes sense to establish a relationship with a single AI-capable vendor that understands the end-to-end data management and curation needs for optimal AI performance.
Easily configurable and scalable localization workflow: The needs of localization buyers vary greatly and are unique to their businesses. Some buyers may only want a quick conversion of critical web content to new target languages and others may need a complete review of all customer-facing content and communications that requires integration with multiple internal systems that have a far-reaching impact on the enterprise IT infrastructure. The ability to handle these two extremes and the many possible options that exist between these two extremes is a highly desirable aspect of an AI-first capability. While simple requirements can be quickly handled by state-of-the-art platform tools that easily blend AI technologies with expert human feedback, more complex requirements may need a more detailed review of specific customer requirements and a process and workflow design that is tailored to unique requirements. However, in all cases, capabilities that allow rapid prototyping and are designed to scale as complexity and scope expand would be very valuable.
End-to-end view of all content and localization data: This would enable data-driven insights to be effectively implemented in upstream processes to improve efficiency and quality outcomes in downstream actions. This comprehensive perspective enables data curation to be done at a maximally benefical level. If AI-first processes have a view of content and data from creation to final translation and delivery across the various delivery platforms used by the enterprise to share and communicate with global customers, the benefits accrue.
A key requirement to enable a global enterprise to be multilingual at scale requires a holistic view of content as it flows through the enterprise. This view is essential not just to be able to process large volumes of content, but also to enable rapid translation turnaround and ensure the trustworthy quality of the transformed content.
The ability to see the transformation of content from creation to final delivery and presentation to global customers allows efficiencies and process streamlining that is not possible in disjointed multi-vendor settings where critical information may be hidden or difficult to access at a global level. An end-to-end view of the data enables systemic improvements in content early in the content lifecycle to make downstream processes more efficient and deliver higher-quality outcomes and output.
As can be seen from the description above, AI-first localization is a data centered and data enabled approach to both large-scale and smaller scale localization where technology combines seamlessly with expert human feedback to produce superior localization outcomes that continuously improve in quality and impact.
The Importance of Data Management & Data Curation
Given that data quality and relevance are such a critical driver of AI-first localization strategies it is worth considering what practices should be followed to ensure the best data. To ensure data quality in machine learning scenarios, several best practices can be followed. These practices help maintain the integrity, accuracy, and reliability of data, which are crucial for developing effective and trustworthy AI models. Here are some key best practices that are today a matter of regular business practice at Translated:
Data Collection and Preprocessing: The data needed for various machine learning tasks is clearly defined and the collection ensures that the data is relevant and comprehensive. The collection process is standardized to ensure uniformity and minimize errors. There are also both automated and human feedback-driven improvements and cleaning being conducted on an ongoing basis. This curation process ensures that the data used by the models is constantly improving and thus yields better outcomes.
Data Annotation and Labeling: Clear guidelines are established so that annotators/post-editors are vetted and consistently provide useful feedback. This is crucial for model improvement and continuing benefits. The feedback from the most expert editors is given more weight to ensure that the models prioritize this feedback.
Data Validation and Monitoring: As these systems are used regularly in production mode there are automated validation rules to ensure that model outputs are continuously validated and corrected if necessary. High-level metrics like Time-to-edit (TTE) are also monitored to ensure that progress is consistent and continuous. These metrics allow problems to be promptly identified and corrected.
Data Governance and Documentation: Establishing a robust data governance framework allows data quality to remain consistently high and relevant metadata related to data sources, projects, transformations, and annotation are maintained to ensure ongoing improvements and audits in case of problems.
Handling Bias and Diversity: Care is taken to use diverse data or very specific data to ensure optimal model generalization and minimal bias. Continuously monitor and mitigate biases in the data to ensure fairness and equity in AI models.
Data Quality Tools and Automation: Use tried and tested tools to automate data cleansing, validation, and monitoring processes. This can include adding real-time data quality checks to maintain high standards throughout the machine learning lifecycle.
By following these best practices, organizations can enhance data quality, leading to more accurate, reliable, and fair machine learning models. These practices also contribute to more efficient AI development processes and better decision-making outcomes.
Data curation and refinement is a continuous and ongoing process and systems are designed to optimize this for different clients in different ways.
For those who have a rigorous data curation process in place, the future of emerging linguistic AI technology will be bright and filled with continuously improving quality and efficiency.